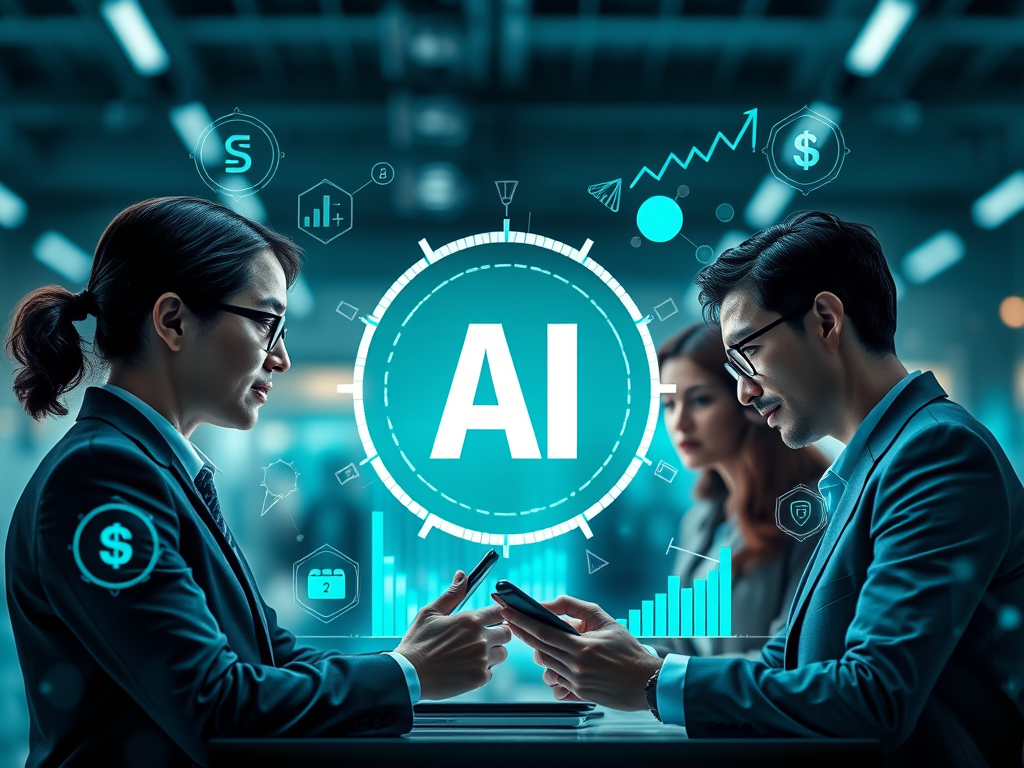
Building a Fair and Explainable Credit Scoring AI: Privacy, Security, and Ethical Principles
Introduction
As artificial intelligence (AI) reshapes the financial industry, credit scoring systems powered by machine learning have become essential for assessing borrower risk. However, these AI-driven models must be designed with fairness, transparency, and security in mind to ensure ethical decision-making. This blog explores the key considerations in developing an explainable and responsible credit scoring AI while complying with privacy, security, and environmental regulations.
Privacy Regulations in Credit Scoring AI
Privacy is paramount when handling sensitive financial and personal data. Various regulations mandate responsible data usage and user rights, including:
Key Privacy Laws and Their Applicability
- General Data Protection Regulation (GDPR – EU): Requires transparency, data minimization, and user rights to explanation and data deletion.
- California Consumer Privacy Act (CCPA – USA): Grants consumers control over their credit data, including opting out of data sales.
- Fair Credit Reporting Act (FCRA – USA): Ensures accurate, fair, and private use of credit data.
- Personal Data Protection Bill (India): Regulates financial data collection and processing, emphasizing consent-based use.
Implementation Strategies
- Data Minimization: Collect only necessary credit-related information.
- User Control: Enable access, correction, and deletion of personal data.
- Anonymization: Use differential privacy to prevent re-identification of individuals.
Security Considerations in AI-driven Credit Scoring
Cyber threats pose significant risks to financial systems, making security a top priority.
Security Challenges & Solutions
- Data Breaches: Encrypt stored credit data (AES-256) and ensure secure data transmission (TLS encryption).
- Unauthorized Access: Implement Role-Based Access Control (RBAC) to restrict data access.
- Fraudulent Applications: Use anomaly detection models to identify suspicious patterns.
- Adversarial Attacks: Protect AI models from manipulation by testing against adversarial inputs.
Environmental Impact of AI in Credit Scoring
Training and deploying machine learning models consume significant energy, raising concerns about AI’s carbon footprint.
Sustainable AI Strategies
- Energy-efficient Models: Optimize algorithms to reduce computational costs.
- Green Data Centers: Use cloud providers committed to renewable energy.
- Batch Processing: Minimize real-time processing to lower energy consumption.
Ethical AI Principles for Credit Scoring
To ensure fairness, trust, and responsible AI use, ethical principles must guide credit scoring models.
1. Transparency & Explainability
Consumers should understand how their credit score is determined.
- Tools Used: SHAP (SHapley Additive Explanations), LIME, and Counterfactual Explanations.
- Visual Dashboards: Provide intuitive breakdowns of credit score factors.
2. Fairness & Bias Mitigation
AI models must avoid discrimination based on race, gender, or socioeconomic status.
- Bias Audits: Detect unfair lending patterns.
- Fairness Constraints: Implement Equalized Odds and Demographic Parity in model training.
3. Accountability & Oversight
AI-driven decisions should be auditable and subject to human review.
- Human-in-the-loop Systems: Allow manual review for disputed credit scores.
- Audit Logs: Maintain a record of AI-generated decisions for regulatory compliance.
4. Privacy & Data Protection
User data should be secure and accessible only for legitimate purposes.
- Data Governance Frameworks: Ensure proper data handling under GDPR/CCPA regulations.
- Opt-in Consent Mechanisms: Allow users to control their financial data.
5. Social Responsibility & Inclusion
AI should enhance financial inclusion, not create barriers.
- Alternative Credit Data: Incorporate rent payments and utility bills for borrowers with no traditional credit history.
- Customizable Models: Adapt credit assessments to diverse economic backgrounds.
Conclusion
Ethical and responsible AI in credit scoring is not just a regulatory requirement—it is a moral imperative. By integrating fairness, privacy, security, and sustainability into AI-driven credit systems, we can build a future where financial inclusion is accessible to all.
Would you like to learn more about how AI can shape the future of ethical lending? Let’s start the conversation! 🚀